Carding 4 Carders
Professional
The Woodpecker tool offers a new approach to the "education" of neural networks.
Researchers from the University of Science and Technology of China (USTC), in collaboration with the Tencent YouTu Lab, have developed a framework called Woodpecker to correct so — called "hallucinations" in multimodal large language models (MLLMs).
MLLMs — Multimodal Large Language Models) are artificial intelligence models that can process and generate information in various formats, mainly text and images. The neural network captures connections between words and visual content, for example, by correlating descriptions with corresponding images, or vice versa.
Hallucinations in MLLMs occur when the text generated by the neural network does not match the image. This problem is becoming more and more urgent, as MLLMs are actively used in various industries: from creating entertainment content to automated customer support systems.
Until now, scientists have solved the problem thoroughly — the model was re-trained on other data, which, of course, required significant computational resources. Woodpecker offers an alternative, less energy-intensive approach.
The new algorithm consists of five stages:
1. The model extracts key ideas from the text.
2. Formulates questions based on the selected concepts.
3. Checks whether the text and image match each other based on visual analysis.
4. Re-describes the images after analyzing your own answers to the questions.
5. Corrects hallucinations based on new introductions.
The name was not chosen by chance: just as a woodpecker "treats" trees, this tool corrects errors in generated materials.
The researchers uploaded Woodpecker's source code to the web so that AI experts could independently evaluate its capabilities. For clarity, the developers also provided an interactive demo of the system, which demonstrates the process of error correction in real time.
Initial experiments were performed on several datasets. With POPE, one of these datasets, the new method increased the accuracy of the base model from 54.67% to 85.33%.
The tool promises to be a real breakthrough in the field of artificial intelligence, and also opens up new horizons for using MLLMs in applications and enterprise programs.
Researchers from the University of Science and Technology of China (USTC), in collaboration with the Tencent YouTu Lab, have developed a framework called Woodpecker to correct so — called "hallucinations" in multimodal large language models (MLLMs).
MLLMs — Multimodal Large Language Models) are artificial intelligence models that can process and generate information in various formats, mainly text and images. The neural network captures connections between words and visual content, for example, by correlating descriptions with corresponding images, or vice versa.
Hallucinations in MLLMs occur when the text generated by the neural network does not match the image. This problem is becoming more and more urgent, as MLLMs are actively used in various industries: from creating entertainment content to automated customer support systems.
Until now, scientists have solved the problem thoroughly — the model was re-trained on other data, which, of course, required significant computational resources. Woodpecker offers an alternative, less energy-intensive approach.
The new algorithm consists of five stages:
1. The model extracts key ideas from the text.
2. Formulates questions based on the selected concepts.
3. Checks whether the text and image match each other based on visual analysis.
4. Re-describes the images after analyzing your own answers to the questions.
5. Corrects hallucinations based on new introductions.
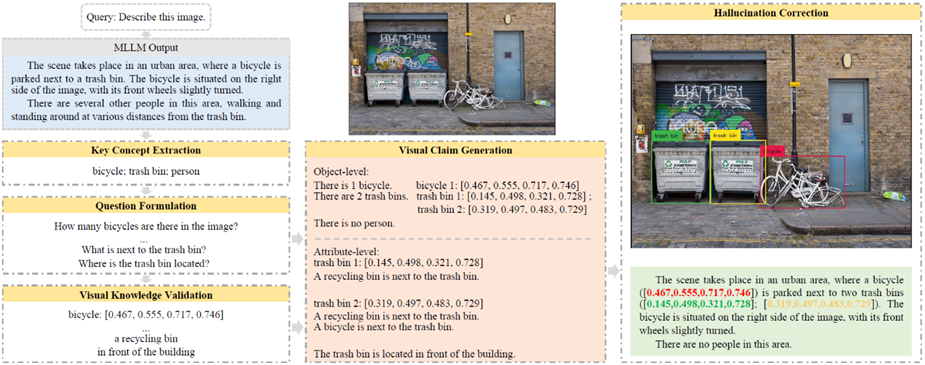
The name was not chosen by chance: just as a woodpecker "treats" trees, this tool corrects errors in generated materials.
The researchers uploaded Woodpecker's source code to the web so that AI experts could independently evaluate its capabilities. For clarity, the developers also provided an interactive demo of the system, which demonstrates the process of error correction in real time.
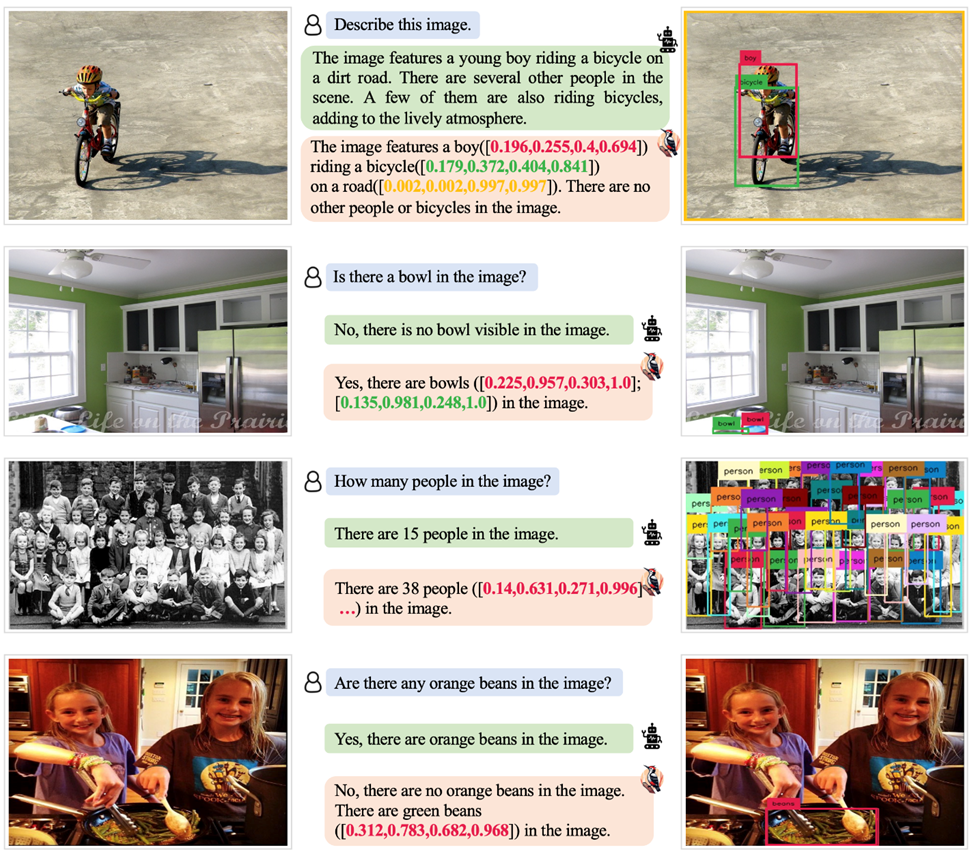
Initial experiments were performed on several datasets. With POPE, one of these datasets, the new method increased the accuracy of the base model from 54.67% to 85.33%.
The tool promises to be a real breakthrough in the field of artificial intelligence, and also opens up new horizons for using MLLMs in applications and enterprise programs.